VOlUME 03 ISSUE 09 September 2024
1Vicente E. Montano, 2Rowena C. Cinco
1,2College of Business Administration Education, University of Mindanao, Bolton St., Davao City (8000) Philippines
DOI : https://doi.org/10.58806/ijsshmr.2024.v3i9n14Google Scholar Download Pdf
ABSTRACT
This research applies the Geometric Brownian Motion-Neural Network (GBM-NN) model to predict the industrial output in the Philippines from January 1993 to December 2022. The GBM-NN model uses the stochastic properties of Geometric Brownian Motion to estimate trends and volatility better and introduces a neural network for more predictive accuracy based on nonlinearity in the data. The result suggests an industrial production series characterized by high variability. Due to the application of the GBM-NN method, it is verified that this model issues a more coherent prediction than traditional methods due to the lower RMSE and better R-square values. Results show a modest recovery in the industrial sector and further illustrate that the model is an excellent tool to help policymakers and industry participants make better decisions during uncertain economic periods.
KEYWORDS:Industrial Output, Geometric Brownian Motion-Neural Network (GBM-NN), Philippines, UN SDG 9
REFERENCES
1) Aheer, A. K., Pradhan, A. K., & Srivastava, R. (2023, July). Application of Feedforward Neural Network in Portfolio Optimization and Geometric Brownian Motion in Stock Price Prediction. In 2023 4th International Conference on Electronics and Sustainable Communication Systems (ICESC) (pp. 1494-1503). IEEE. https://doi.org/10.1109/ICESC57686.2023.10193046
2) Asarin, E., Dang, T., & Girard, A. (2007). Hybridization methods for the analysis of nonlinear systems. Acta Informatica, 43, 451-476.
3) Asian Development Bank. (2008). Philippines: Critical Development Constraints. https://www.adb.org/sites/default/files/publication/29274/cdc-philippines.pdf
4) Azizah, M., Irawan, M. I., & Putri, E. R. M. (2020, June). Comparison of stock price prediction using geometric Brownian motion and multilayer perceptron. In AIP Conference Proceedings (Vol. 2242, No. 1). AIP Publishing. https://doi.org/10.1063/5.0008066
5) Blechschmidt, J., & Ernst, O. G. (2021). Three ways to solve partial differential equations with neural networks — A review. GAMM-Mitteilungen, 44(2). https://doi.org/10.1002/gamm.202100006
6) Briguglio, L., Cordina, G., Farrugia, N., & Vella, S. (2014). Economic vulnerability and resilience: concepts and measurements. In Measuring Vulnerability in Developing Countries (pp. 47-65). Routledge.
7) Bruno, G., & Lupi, C. (2004). Forecasting industrial production and the early detection of turning points. Empirical Economics, 29(3). https://doi.org/10.1007/s00181-004-0203-y
8) Burchell, B. (2009). Flexicurity as a moderator of the relationship between job insecurity and psychological well-being. Cambridge Journal of Regions, Economy and Society, 2(3), 365-378.
9) Debuque-Gonzales, M. (2021). Navigating the COVID-19 storm: Impact of the pandemic on the Philippine economy and macro responses of government (No. 2021-39). PIDS Discussion Paper Series.
10) Dong, W., Yao, J., & Zhang, B. (2022). Quantitative Evaluation and Characteristic Analysis of Resource Allocation Efficiency of the Energy Industry in the Yangtze River Economic Belt. Sustainability, 14(24), 16971. https://doi.org/10.3390/su142416971
11) Intal Jr, P. S., Borromeo, M. R. V., & Castillo, J. C. E. D. (2008). Sustaining the Philippine manufacturing sector. Philippine Review of Economics (Online ISSN 2984-8156), 45(1).
12) Jean-Paul, F., & Martine, D. (2018). Beyond GDP measuring what counts for economic and social performance: measuring what counts for economic and social performance. OECD Publishing.
13) Jongbo, O. C. (2014). The impact of real exchange rate fluctuation on industrial output in Nigeria. Transport, 2(2.4), 3-1.
14) Joshua, T., Isah, A., Ibrahim, A., Abdullahi, U., & Danyaro, M. L. (2023). COMPARATIVE ANALYSIS OF GEOMETRIC BROWNIAN MOTION, ARTIFICIAL NEURAL NETWORK AND NAIVE BAYESIAN TECHNIQUES USING NIGERIA STOCK EXCHANGE DATA. FUDMA JOURNAL OF SCIENCES, 7(5), 258-265.
15) Kholodilin, K. A., Thomas, T., & Ulbricht, D. (2014). Do media data help to predict German industrial production?. https://dx.doi.org/10.2139/ssrn.2459780
16) Kühn, R., & Neu, P. (2008). Intermittency in an interacting generalization of the geometric Brownian motion model. Journal of Physics A: Mathematical and Theoretical, 41(32), 324015. https://doi.org/10.1088/1751-8113/41/32/324015
17) McNichols, J. P., & Rizzo, J. L. (2012). Stochastic GBM methods for modeling market prices. In Casualty Actuarial Society E-Forum, Summer 2012.
18) Noy, I., & Yonson, R. (2016). A survey of the theory and measurement of economic vulnerability and resilience to natural hazards. https://ir.wgtn.ac.nz/handle/123456789/19394
19) O’Neill, A. (2023). Philippines - Employment by economic sector. Statista. https://www.statista.com/statistics/578788/employment-by-economic-sector-in-philippines/
20) Palley, T. I. (2002). Keynesian macroeconomics and the theory of economic growth: putting aggregate demand back in the picture. The Economics of Demand-Led Growth: Challenging the Supply-Side Vision of the Long Run, 19-40. https://doi.org/10.4337/9781843765325
21) Petropoulos, F. (2022). Forecasting: Theory and practice. International Journal of Forecasting, 38(3). sciencedirect. https://doi.org/10.1016/j.ijforecast.2021.11.001
22) Philippines – Preserving economic stability, financing development and anticipating climate issues. (2024). Www.afd.fr. https://www.afd.fr/en/ressources/philippines-preserving-economic-stability-financing-development-and-anticipating-climate-issues
23) Philippine Statistics Authority, Republic of the Philippines. (2024). Psa.gov.ph. https://psa.gov.ph/statistics/national-accounts/sector/Industry#:~:text=In%20terms%20of%20its%20contribution
24) Philippine Statistics Authority. (2023). Labor Force Survey | Philippine Statistics Authority | Republic of the Philippines. Psa.gov.ph. https://psa.gov.ph/statistics/labor-force-survey
25) Philippines: Toward Sustainable and Rapid Growth: Recent Developments and the Agenda Ahead--IMF Occasional Paper No. 187. (n.d.). Www.imf.org. https://www.imf.org/external/pubs/nft/op/187/
26) Pineda, J., Midtvedt, B., Bachimanchi, H., Noé, S., Midtvedt, D., Volpe, G., & Manzo, C. (2023). Geometric deep learning reveals the spatiotemporal features of microscopic motion. Nature Machine Intelligence, 5(1), 71-82. https://doi.org/10.1038/s42256-022-00595-0
27) Pitelis, C. N. (2018). Supply-side Strategy for Productivity, Competitiveness and Convergence for the EU and the CEECs. In Foreign direct investment in Central and Eastern Europe (pp. 203-229). Routledge.
28) Sankaran, G., Sasso, F., Kepczynski, R., & Chiaraviglio, A. (2019). Improving Forecasts with Integrated Business Planning. Springer.
29) Sadeqi, H., Fadaeinejad, M., & Varzideh, A. (2019). Application of Geometric Brownian motion in prediction of gold price and currency rate. Journal of Investment Knowledge, 8(30), 251-270.
30) Tuovila, A. (2020, May 27). Forecasting. Investopedia. https://www.investopedia.com/terms/f/forecasting.asp
31) Vidmer, A., Zeng, A., Medo, M., & Zhang, Y. C. (2015). Prediction in complex systems: The case of the international trade network. Physica A: Statistical Mechanics and its Applications, 436, 188-199.
32) Web Publisher. (2021, March 16). PH logistics stakeholders identify 2021 industry prospects, challenges - PortCalls Asia. PortCalls Asia. https://www.portcalls.com/ph-logistics-stakeholders-identify-2021-industry-prospects-challenges/
33) Wieland, V., & Wolters, M. (2013, January 1). Chapter 5 - Forecasting and Policy Making (G. Elliott & A. Timmermann, Eds.). ScienceDirect; Elsevier. https://www.sciencedirect.com/science/article/abs/pii/B9780444536839000050
34) World Bank. (2024). World Bank Open Data. World Bank. https://data.worldbank.org/
35) Ying, C.-M. (2023, August 30). Optimizing Demand Forecasting & Supply Planning» New Horizon. New Horizon. https://www.newhorizon.ai/blogs/whats-the-value-of-demand-forecasting/#:~:text=%E2%80%9CImprov%5Bing%5D%20forecasting%20accuracy
36) Yu, C., Fu, C., & Xu, P. (2024). Energy shock, industrial transformation and macroeconomic fluctuations. International Review of Financial Analysis, 92, 103069. https://doi.org/10.1016/j.irfa.2024.103069
37) Zhan, J., & Karl, J. (2016). Investment incentives for sustainable development. In Rethinking investment incentives: Trends and policy options (pp. 204-227). Columbia University Press.
38) Zohuri, B., Rahmani, F. M., & Behgounia, F. (2022). Knowledge is power in four dimensions: models to forecast future paradigm: with artificial intelligence integration in energy and other use cases. Academic Press.
VOlUME 03 ISSUE 09 SEPTEMBER 2024
Indexed In
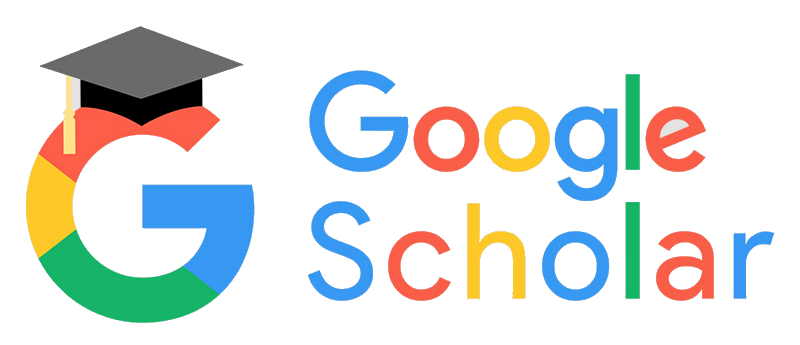